AI for Natural Product Drug Discovery
Bacteria, fungi, plants, and animals produce a variety of specialized metabolites, including peptides, polyketides, sugars, terpenes, and alkaloids. These natural products play crucial roles in complex interorganism interactions, acting as signals, weapons, nutrient scavengers, and stress protectors. Although previously commonly used as antibiotics, chemotherapeutics, immunosuppressants, and crop protection agents, natural products have become less popular in industry in recent years than before due to the rise of combinatorial chemistry and high-throughput screening.
The genes for most metabolite biosynthetic pathways in bacteria and fungi (and some plants and animals) occur as clusters in the genomes of the producing organisms: more than 2,500 of these biosynthetic gene clusters (BGCs) and their products have now been experimentally identified Characterized. This physical clustering has the potential to facilitate the identification of millions of putative new molecular biosynthetic pathways through computational genome analysis, providing a starting point for drug discovery.
AI is currently being used to predict the chemical structure of BGC products based on DNA sequences, and key training data can be obtained through known biosynthetic pathways and their natural products. However, there is an urgent need for more efficient methods to filter and prioritize the large predicted biosynthetic diversity of natural products to identify drug leads.
Figure 1: Applications of artificial intelligence in natural product and drug discovery.
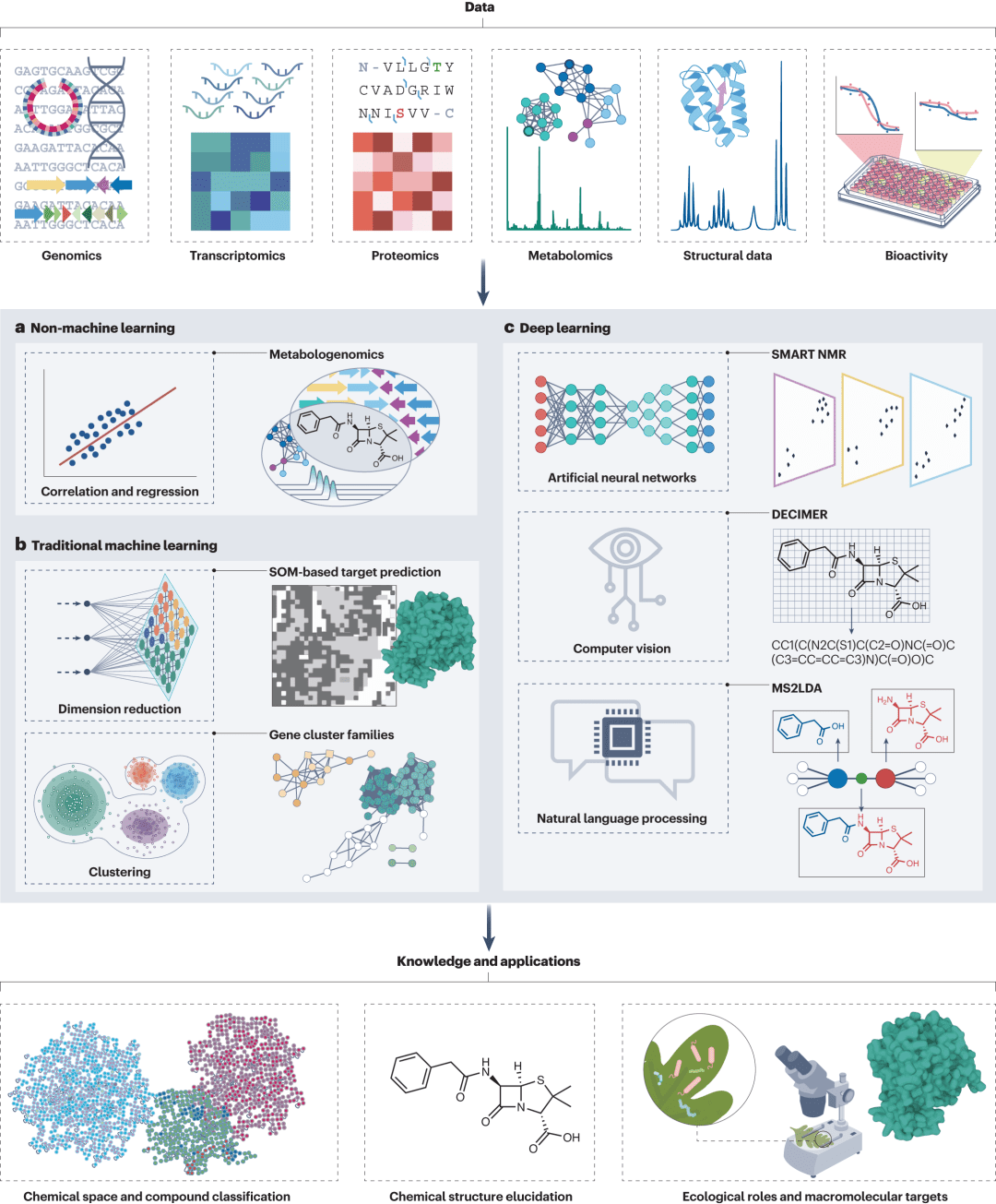
Figure 2: Example of natural product molecules discovered using AI.
Including using the chemprop algorithm to discover the new antibiotic Halicin; using a convolutional neural network to predict the structures of rivulariapeptolides and symplocolide A from complex microbial extracts; using SVM to discover Prstinin A3 by mining whole-genome information.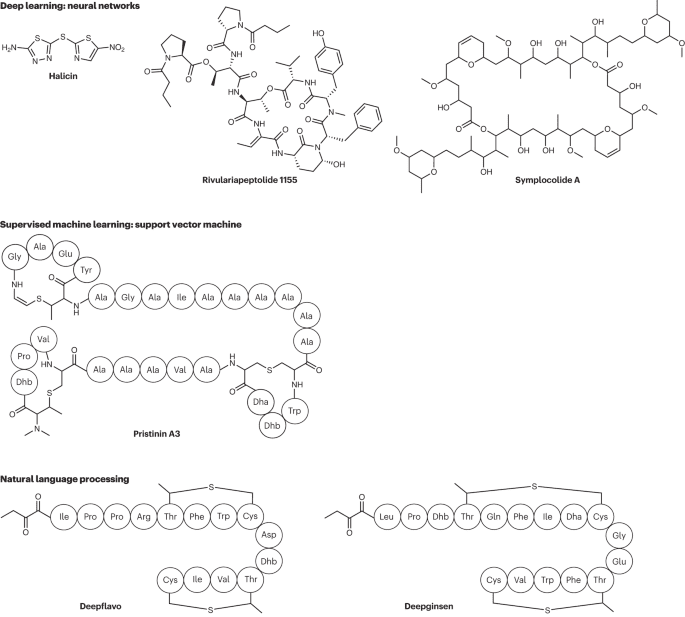
Figure 3: Prediction of bioactive and macromolecular targets based on genomic, metabolomic, and phenotypic data.
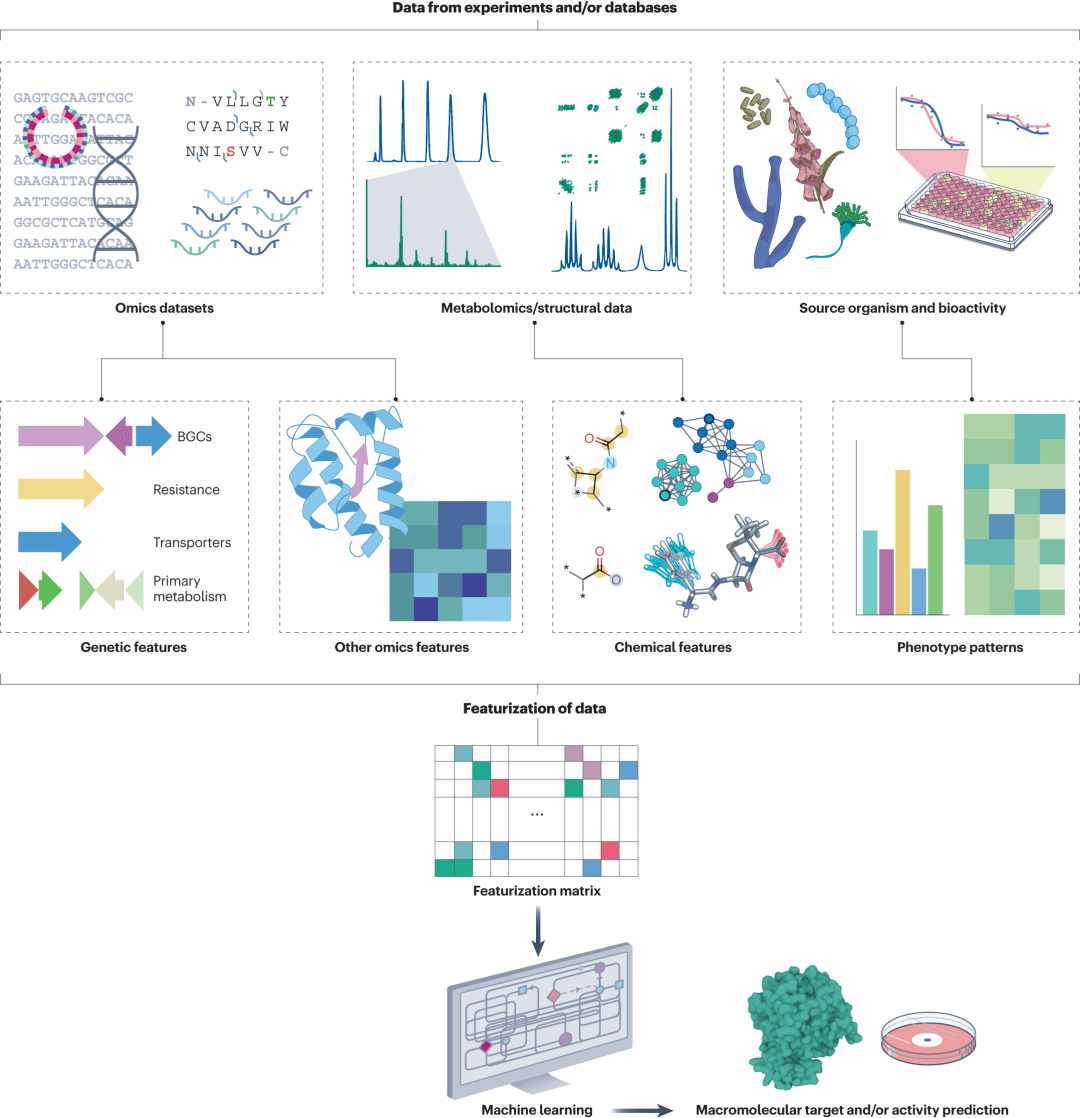
Figure 4: Molecular characterization of commonly used natural products, including pharmacophore, molecular fingerprint, SMILES, 3D dynamics and intermolecular interactions.
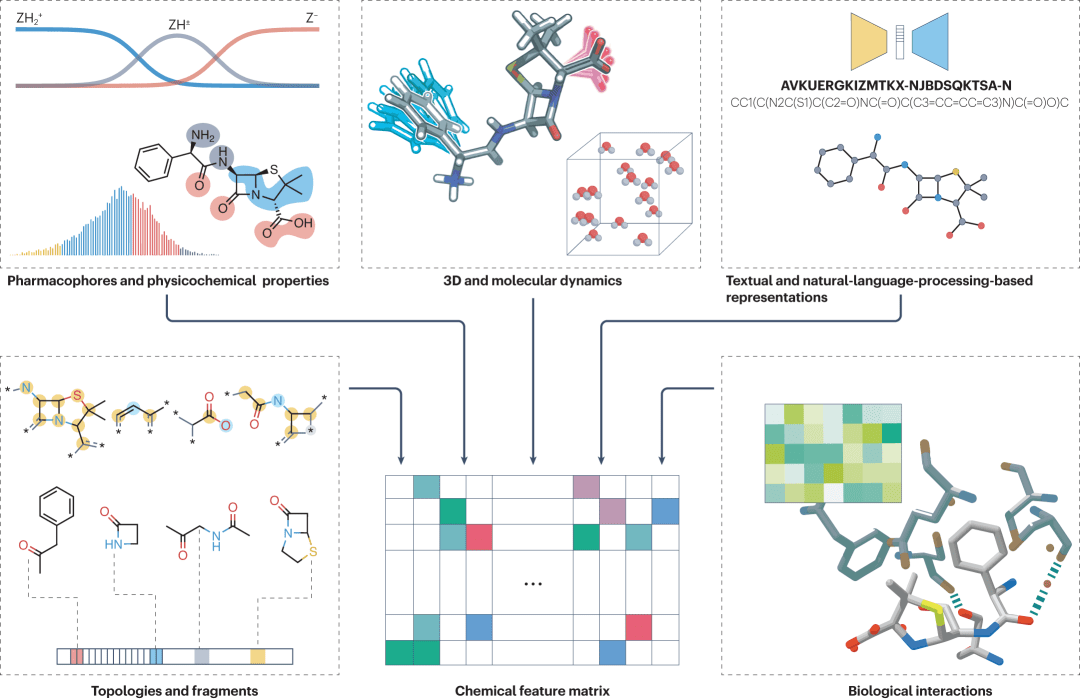
Figure 5: Storing and sharing natural product data: infrastructure and incentives.
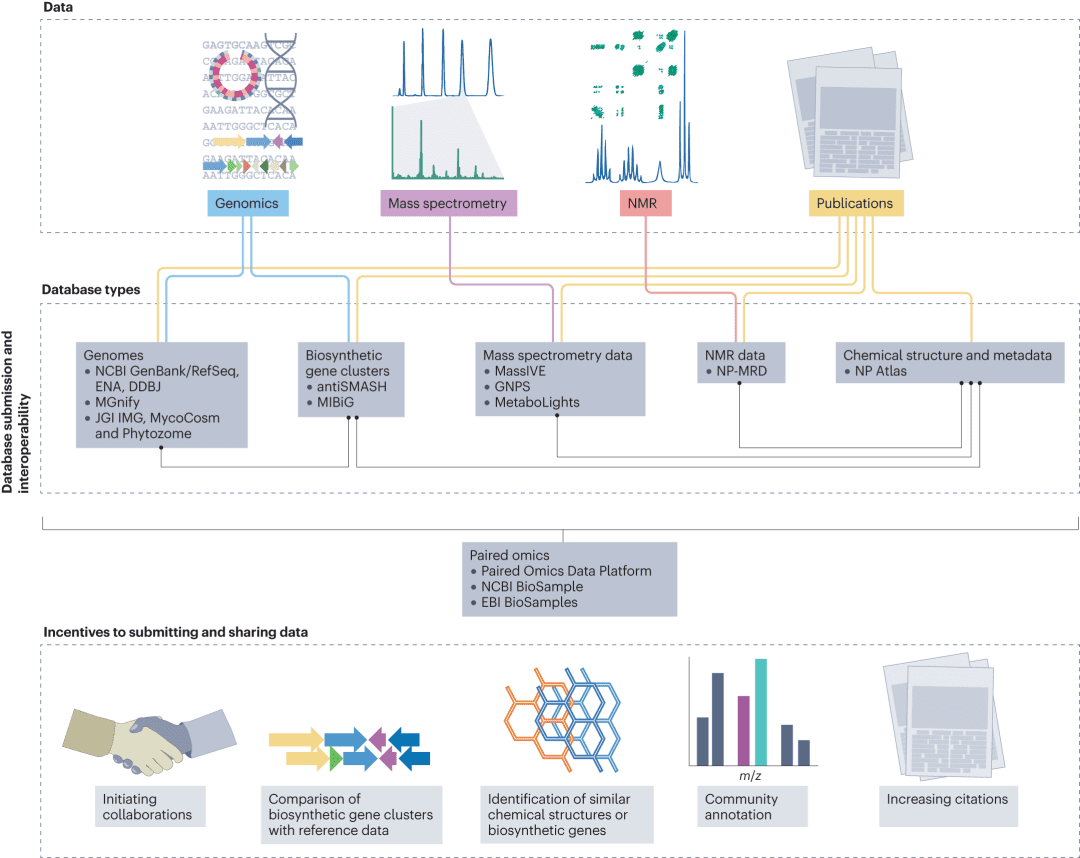